Category-Level 6D Object Pose Estimation via Cascaded Relation and Recurrent Reconstruction Networks
CSE, The Chinese University of Hong Kong1
T Stone Robotics Institute, The Chinese University of Hong Kong2
International Conference on Intelligent Robots and Systems(IROS) 2021
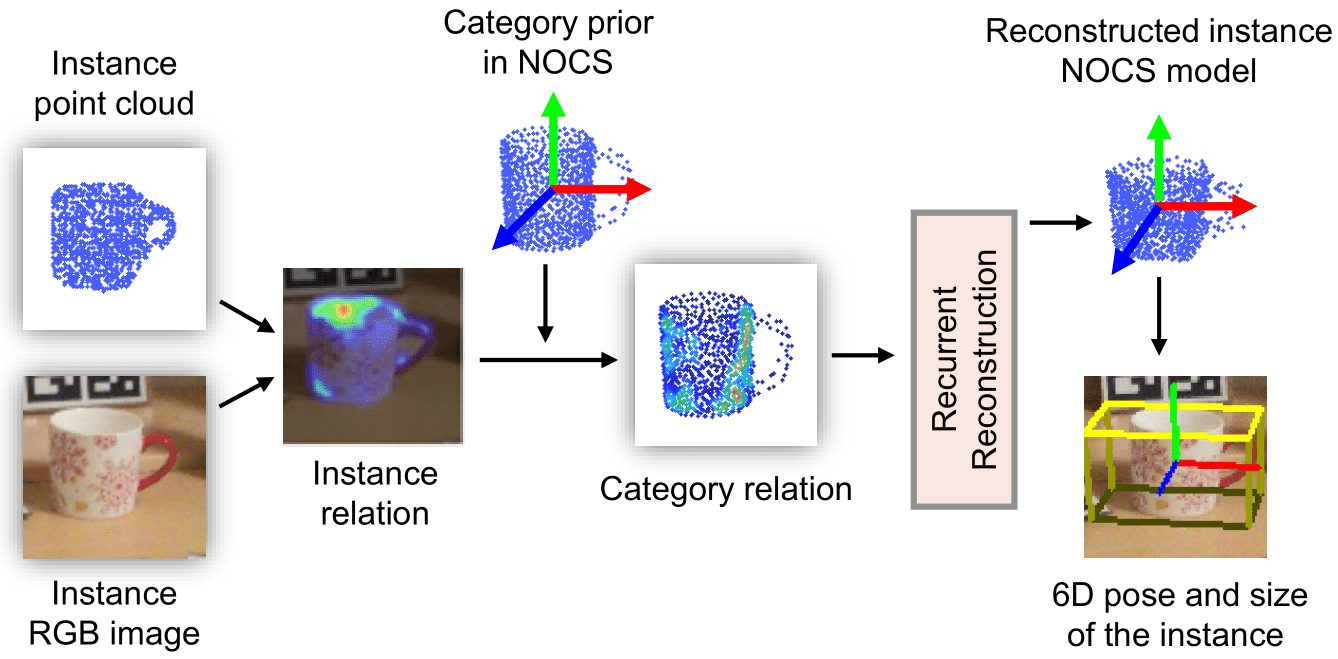
Overview
Category-level 6D pose estimation, aiming to predict the location and orientation of unseen object instances, is fundamental to many scenarios such as robotic manipulation and augmented reality, yet still remains unsolved.
Precisely recovering instance 3D model in the canonical space and accurately matching it with the observation is an essential point when estimating 6D pose for unseen objects. In this paper, we achieve accurate category-level 6D pose estimation via cascaded relation and recurrent reconstruction networks. Specifically, a novel cascaded relation network is dedicated for advanced representation learning to explore the complex and informative relations among instance RGB image, instance point cloud and category shape prior. Furthermore, we design a recurrent reconstruction network for iterative residual refinement to progressively improve the reconstruction and correspondence estimations from coarse to fine. Finally, the instance 6D pose is obtained leveraging the estimated dense correspondences between the instance point cloud and the reconstructed 3D model in the canonical space. We have conducted extensive experiments on two well-acknowledged benchmarks of category-level 6D pose estimation, with significant performance improvement over existing approaches.
Introduction Video
Citation
@inproceedings{wang2021category, title={Category-Level 6D Object Pose Estimation via Cascaded Relation and Recurrent Reconstruction Networks}, author={Wang, Jiaze and Chen, Kai and Dou, Qi}, booktitle = {2021 IEEE/RSJ International Conference on Intelligent Robots and Systems (IROS)}, year={2021} }